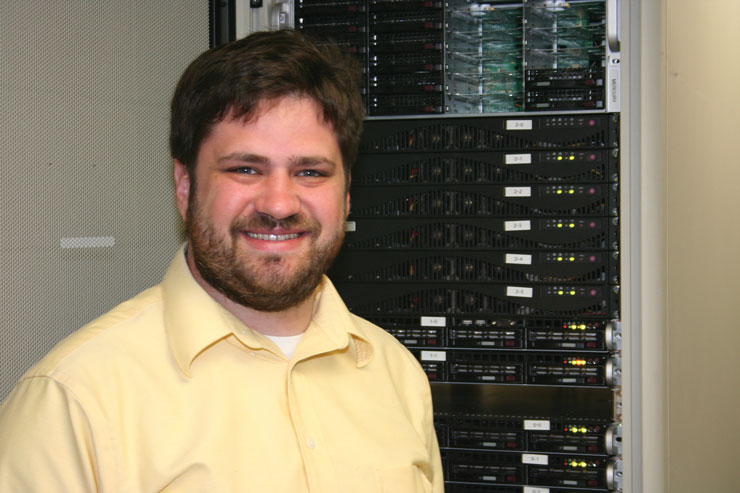
Retinitis pigmentosa is a unique eye disease that requires a unique computational approach.
About 100,000 people in the United States have the condition, which features damage to the retina and can results in blindness. More than 180 retinal disease genes have been identified in approximately 50 percent of observed cases.
Alex Wagner, a Ph.D. student in the Interdisciplinary Graduate Program in Genetics at the University of Iowa, has created a computer algorithm that integrates publicly available online genomic data sets into a machine-learning model to prioritize genes identified in patients with retinal disease, including retinitis pigmentosa.
The research was published in the June 2013 edition of the journal Human Mutation.
“I’m trying to identify those genes that are responsible for retinitis pigmentosa in families,” says Wagner, first author of the study who specializes in computational genetics. “If we can identify those genes, the next step is to attempt to correct those genes in the eye. Clinical trials at Iowa have already demonstrated the approach to correct one such gene in the eye. We can take a retrovirus that has a healthy copy of your gene and give an injection in the eye.”
Wagner’s novel algorithm is titled PULP—positive and unlabeled learning for prioritization. Wagner generated a set of computational vectors of data sets from human retina and mouse retina to observe patterns in gene expression in tissues of the body and eye.
Wagner’s algorithm tests 17,147 genes and calculates probabilities that any gene is a member of a known disease gene set. In comparison to ENDEAVOUR, his results demonstrated a marked improvement in retinal disease gene prioritization using expert-selected data versus general data sets. ENDEAVOUR is a software application for gene prioritization in multiple species.
Genes known to be associated with retinal disease mutations were extracted from RetNet, a manually curated online list of such genes. Wagner and his expert colleagues at the UI selected 13 candidate lists from previous studies to observe algorithm performance.
Wagner’s algorithm found that in 54 percent of these cases, the highest ranked gene was a RetNet gene, and in 77 percent of cases a RetNet gene was within the top five ranked genes. Comparatively, ENDEAVOUR predicted a RetNet gene as the most likely candidate in eight percent of cases and predicted a RetNet gene within the top five in 38 percent of cases.
“My approach allows us to look at a genetic system and many explanatory variables simultaneously to try and develop a machine learning model that can predict whether or not another gene could cause the disease,” says Wagner, a graduate research assistant in the Coordinated Laboratory for Computational Genomics. “We’ve looked at several previous studies that have identified genes that cause these diseases and we’ve shown that this process does select for those genes very significantly.
“This is another line of evidence. It’s not a green flag or red flag sort of thing. This is a prioritization strategy.”
Wagner needs a day to run data on the University of Iowa Helium computer cluster. He is hoping to reduce the time to 20 minutes or less on a desktop machine for multiple-model testing. Wagner and his colleagues are using the output from his technique to identify new disease genes.
According to Wagner, the algorithm is broadly applicable to any heterogeneous disease class, as well as complex diseases such as certain cancers or age-related macular degeneration.
“A similar technique with different data sets can be used to try and isolate genes that are similar to the ones already known,” Wagner says. “The only limiting factor of my algorithm is the expert knowledge required to grab the correct data sets.”
Wagner presented his research at the 21st Annual International Conference on Intelligent Systems for Molecular Biology (ISMB) in Berlin, Germany, in July. He received a travel grant from the National Science Foundation to attend the conference.
NIH Predoctoral Fellow
Wagner, who earned a B.S. in biology at Iowa State in 2008, has been recognized for his academic excellence at the UI.
Born in Long Beach, Calif., Wagner is a National Institutes of Health Predoctoral Fellow who is funded by institutional training grants in both bioinformatics and genetics. A predoctoral fellowship is the most prestigious honor given by the NIH to graduate students.
“For me, it’s an opportunity. Being awarded this fellowship means I can dedicate more of my time to my research,” Wagner says. “That allows me to focus on the research that I find most important.”